What Is The Maximum Number Of Principal Components For Optimal Dimensionality Reduction?
Statquest: Principal Component Analysis (Pca), Step-By-Step
Keywords searched by users: What is the maximum number of principal components all principal components are orthogonal to each other, pca all principal components are orthogonal to each other, how to choose principal components, what is the assumption made by principal component analysis (pca)?, PCA choose number of components, principal component analysis for dummies, principal component analysis numerical example, higher value of k means more regularization
How Many Principal Components Are Possible?
The number of principal components that can be computed in a dataset depends on a minimum of two factors: the number of observations (n) and the number of variables (p) in the dataset. Specifically, the maximum number of principal component loadings that can be calculated is the lesser of (n-1) and p.
To illustrate this concept, let’s consider an example with the first 4 principal components and the first 5 rows of data. It’s important to note that when calculating the principal component score vector, there’s no need to perform the multiplication of the loadings with the data. This simplifies the process and helps in dimensionality reduction, making data analysis more manageable.
For instance, as of March 21, 2016, this fundamental understanding of principal component analysis is crucial for effectively reducing the dimensionality of datasets and extracting meaningful patterns and information.
What Should The Number Of Principal Components Pca Be Equal To?
What should the number of principal components in Principal Component Analysis (PCA) equal to? The number of principal components in PCA should be less than or equal to the number of original variables. This fundamental concept relates to the total variation present in a given dataset. In PCA, the primary objective is to discern the principal components, which are directions within the data where the variation is maximized. This technique allows for dimensionality reduction while retaining the essential information contained within the original dataset.
What Are The Limitations Of Principal Component Analysis?
Understanding the Constraints of Principal Component Analysis (PCA)
Principal Component Analysis (PCA) is undeniably a potent and adaptable analytical tool. However, like any methodology, it does come with its set of limitations that are crucial to acknowledge for a more comprehensive grasp of its applicability. One noteworthy limitation is PCA’s sensitivity to variable selection and the order in which observations are arranged. This means that altering the choice of variables or rearranging your data may yield disparate outcomes. Therefore, when employing PCA, it is vital to consider how the initial data configuration and variable choices can influence the final results. By recognizing these constraints, researchers can make more informed decisions when utilizing PCA for data analysis.
Update 47 What is the maximum number of principal components
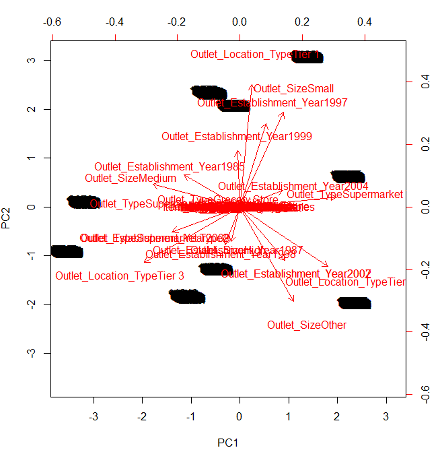
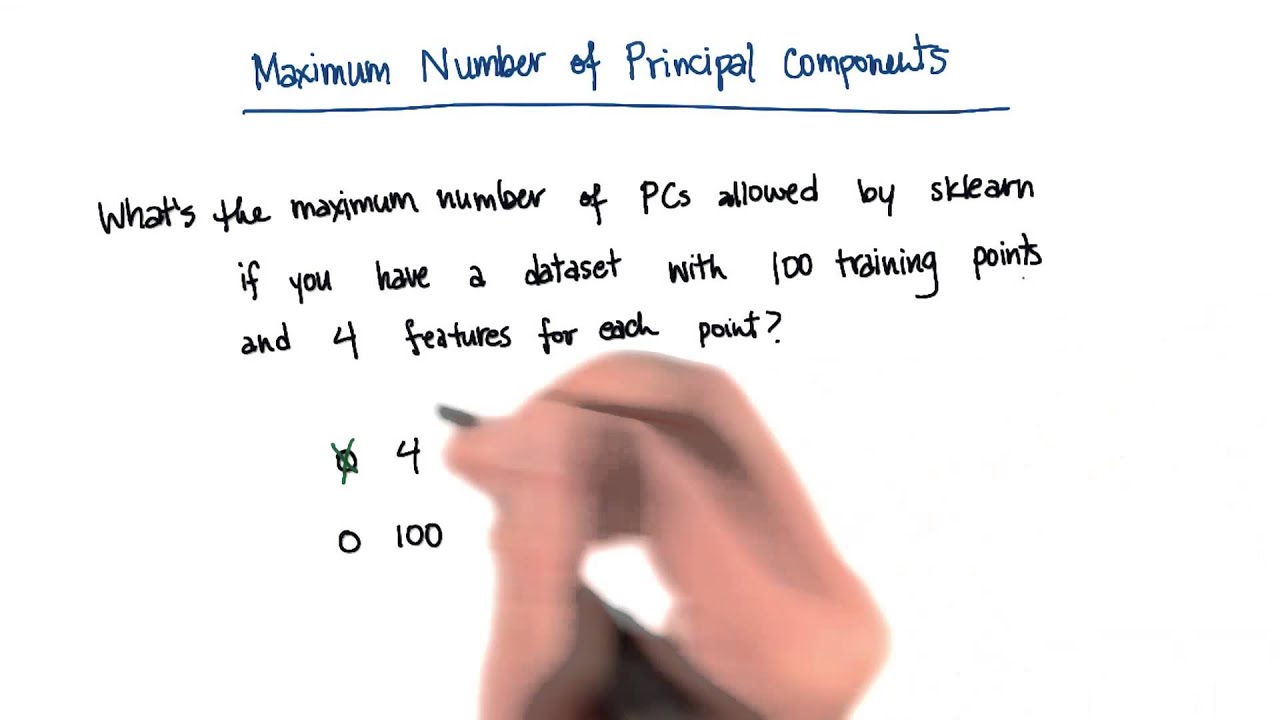
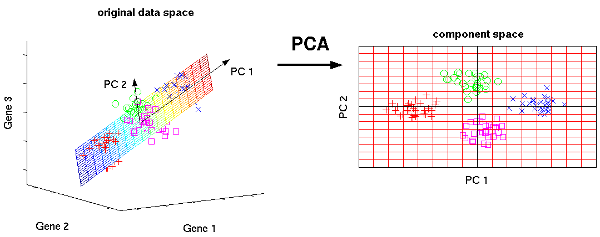
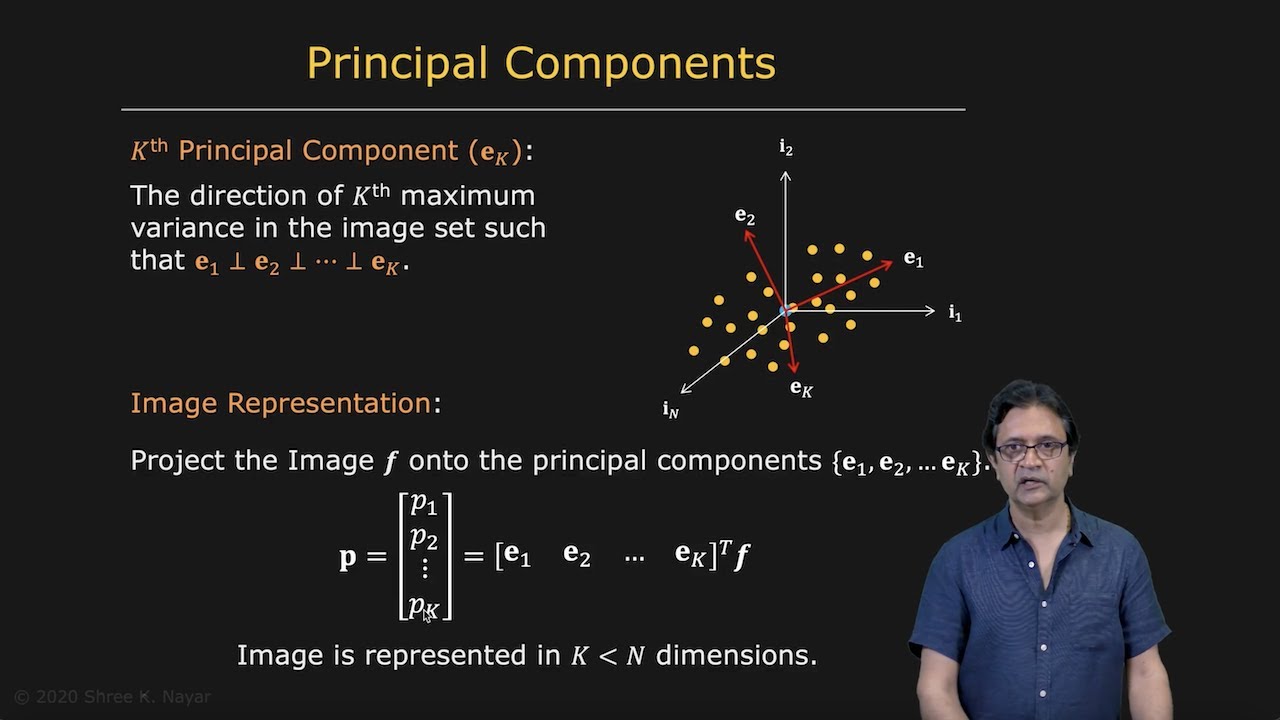

Categories: Details 36 What Is The Maximum Number Of Principal Components
See more here: b1.brokengroundgame.com
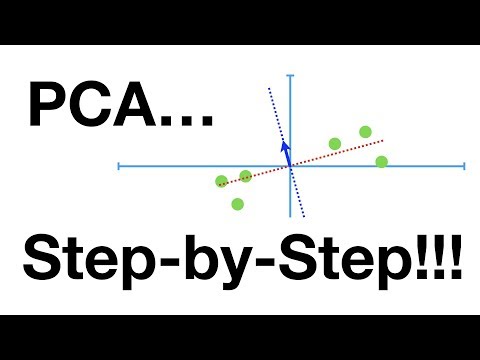
It’s based on the number of features. If you had a dataset with 10 features then the maximum number of principal components is 10. Note that it often takes far fewer than the max number of PCs to explain most of the variance in the dataset.The maximum number of principal component loadings in a data set is a minimum of (n-1, p). Let’s look at the first 4 principal components and first 5 rows. 3. To compute the principal component score vector, we don’t need to multiply the loading with data.The number of principal components is less than or equal to the number of original variables. The information in a given data set corresponds to the total variation it contains. The goal of PCA is to identify directions (or principal components) along which the variation in the data is maximal.
Learn more about the topic What is the maximum number of principal components.
- What is the maximum number of principal components … – Quora
- Principal Component Analysis | PCA Explained
- PCA – Principal Component Analysis Essentials – Articles – STHDA
- Challenges and Limitations of Using PCA for Data Analysis – LinkedIn
- What does it mean for all principal components to capture a small …
- How Many Principal Components to Take in PCA? – Baeldung
See more: blog https://b1.brokengroundgame.com/media